Gradient-Domain Volumetric Photon Density Estimation
Adrien Gruson, The University of Tokyo, JFLI, Japan
Binh-Son Hua, The University of Tokyo, Japan
Nicolas Vibert, McGill University, Canada
Derek Nowrouzezahrai, McGill University, Canada
Toshiya Hachisuka, The University of Tokyo, Japan
In ACM Trans. Graph. (SIGGRAPH), 2018.
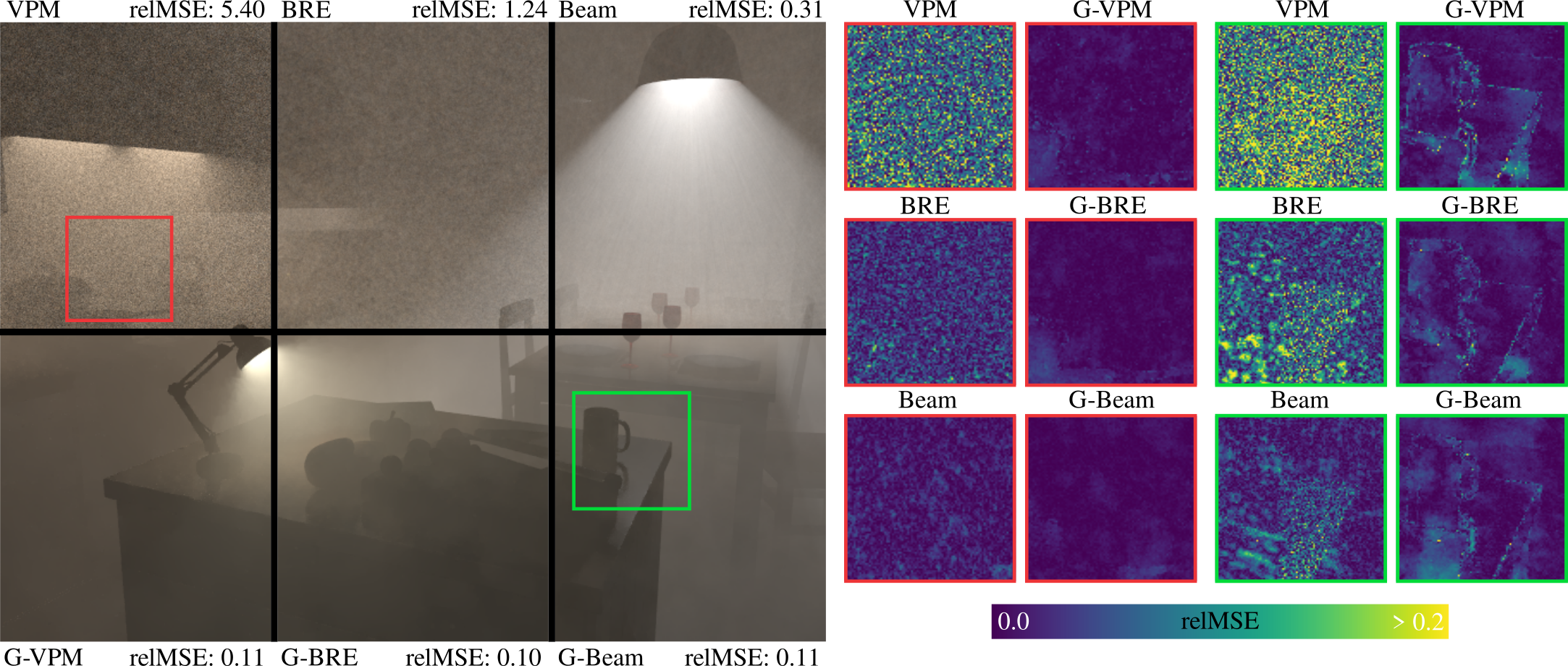
Abstract
Gradient-domain rendering can improve the convergence of surface-based light transport by exploiting smoothness in image space. Scenes with participating media exhibit similar smoothness and could potentially benefit from gradient-domain techniques. We introduce the first gradient-domain formulation of image synthesis with homogeneous participating media, including four novel and efficient gradient-domain volumetric density estimation algorithms. We show that naive extensions of gradient domain path-space and density estimation methods to volumetric media, while functional, can result in inefficient estimators. Focussing on point-, beam- and plane-based gradient-domain estimators, we introduce a novel shift mapping that eliminates redundancies in the naive formulations using spatial relaxation within the volume. We show that gradient-domain volumetric rendering improve convergence compared to primal domain state-of-the-art, across a suite of scenes. Our formulation and algorithms support progressive estimation and are easy to incorporate atop existing renderers.
Downloads
Publication
- Paper (author's version), PDF
- Publisher's official version – external link, may require a subscription
Code & Data
Presentation & Video
Additional
Cite
Adrien Gruson, Binh-Son Hua, Nicolas Vibert, Derek Nowrouzezahrai, and Toshiya Hachisuka . Gradient-Domain Volumetric Photon Density Estimation, ACM Trans. Graph. (SIGGRAPH), 2018.@article{Gruson:2018:GradientVPM, title={Gradient-domain volumetric photon density estimation}, author={Gruson, Adrien and Hua, Binh-Son and Vibert, Nicolas and Nowrouzezahrai, Derek and Hachisuka, Toshiya}, journal={ACM Transactions on Graphics (TOG)}, volume={37}, year={2018}, doi={10.1145/3197517.3201363} }
Copy to clipboard