Deep Adaptive Wavelet Network
Maria Ximena Bastidas Rodriguez, National University of Colombia, Colombia
Adrien Gruson, McGill University, Canada
Luisa F. Polania, Target Corporation, USA
Shin Fujieda, AMD, Japan
Flavio Prieto Ortiz, National University of Colombia, Colombia
Kohei Takayama, Digital Frontier Inc., Japan
Toshiya Hachisuka, The University of Tokyo, Japan
In WACV, 2020.
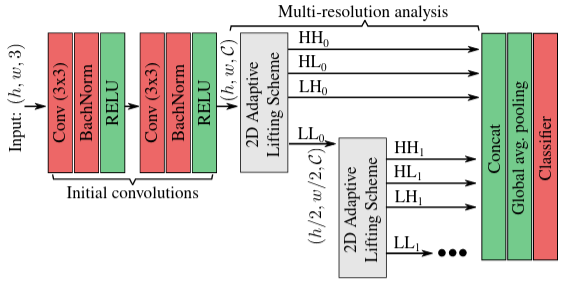
Abstract
Even though convolutional neural networks have become the method of choice in many fields of computer vision, they still lack interpretability and are usually designed manually in a cumbersome trial-and-error process. This paper aims at overcoming those limitations by proposing a deep neural network, which is designed in a systematic fashion and is interpretable, by integrating multiresolution analysis at the core of the deep neural network design. By using the lifting scheme, it is possible to generate a wavelet representation and design a network capable of learning wavelet coefficients in an endto-end form. Compared to state-of-the-art architectures, the proposed model requires less hyper-parameter tuning and achieves competitive accuracy in image classification tasks. The Code implemented for this research is available at https://github.com/mxbastidasr/DAWN_WACV2020.
Downloads
Publication
- Paper (author's version), PDF
- Publisher's official version – external link, may require a subscription
Code & Data
Cite
Maria Ximena Bastidas Rodriguez, Adrien Gruson, Luisa F. Polania, Shin Fujieda, Flavio Prieto Ortiz, Kohei Takayama, and Toshiya Hachisuka . Deep Adaptive Wavelet Network, WACV, 2020.@inproceedings{Rodriguez:2020:DAWN, title={Deep Adaptive Wavelet Network}, author={Rodriguez, Maria Ximena Bastidas and Gruson, Adrien and Polania, Luisa and Fujieda, Shin and Prieto, Flavio and Takayama, Kohei and Hachisuka, Toshiya}, booktitle={The IEEE Winter Conference on Applications of Computer Vision}, year={2020}, doi={10.1109/WACV45572.2020.9093580} }
Copy to clipboard